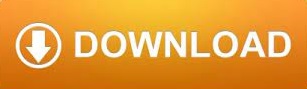
Defining the governance and transformation layer Data lakes on Amazon S3 also integrate with other AWS ecosystem services (for example, AWS Athena for interactive querying or third-party tools running off Amazon Elastic Compute Cloud (Amazon EC2) instances). The data lake layer provides 99.999999999% data durability and supports various data formats, allowing you to future proof the data lake. AWS Database Migration Service (AWS DMS) connects and migrates data from relational databases, data warehouses, and NoSQL databases.įigure 4.AWS Glue connects to real-time data streams to extract, load, transform, clean, and enrich data.This service is for systems that support batch transfer modes and have no real-time requirements, such as external data entities. AWS Transfer Family for SFTP integrates with source systems to extract data using secure shell (SSH), SFTP, and FTPS/FTP.Data is then placed into a data lake.įigure 3 shows the following services to be included in this layer: Services in this layer work directly with the source systems based on their supported data extraction patterns. This can happen while services in the purpose-built consumption layer address individual business unit requirements. Services can be added, removed, and updated independently when new data sources are identified like data sources to enrich data via AWS Data Exchange. This lake house architecture provides you a de-coupled architecture.
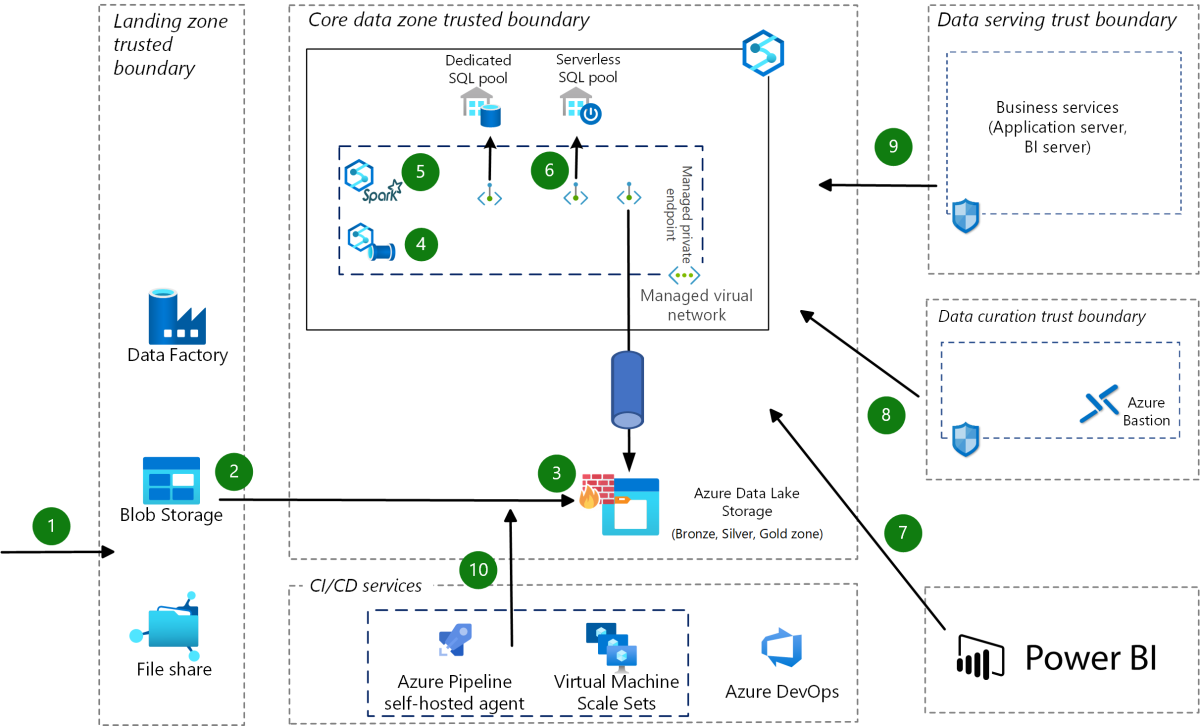
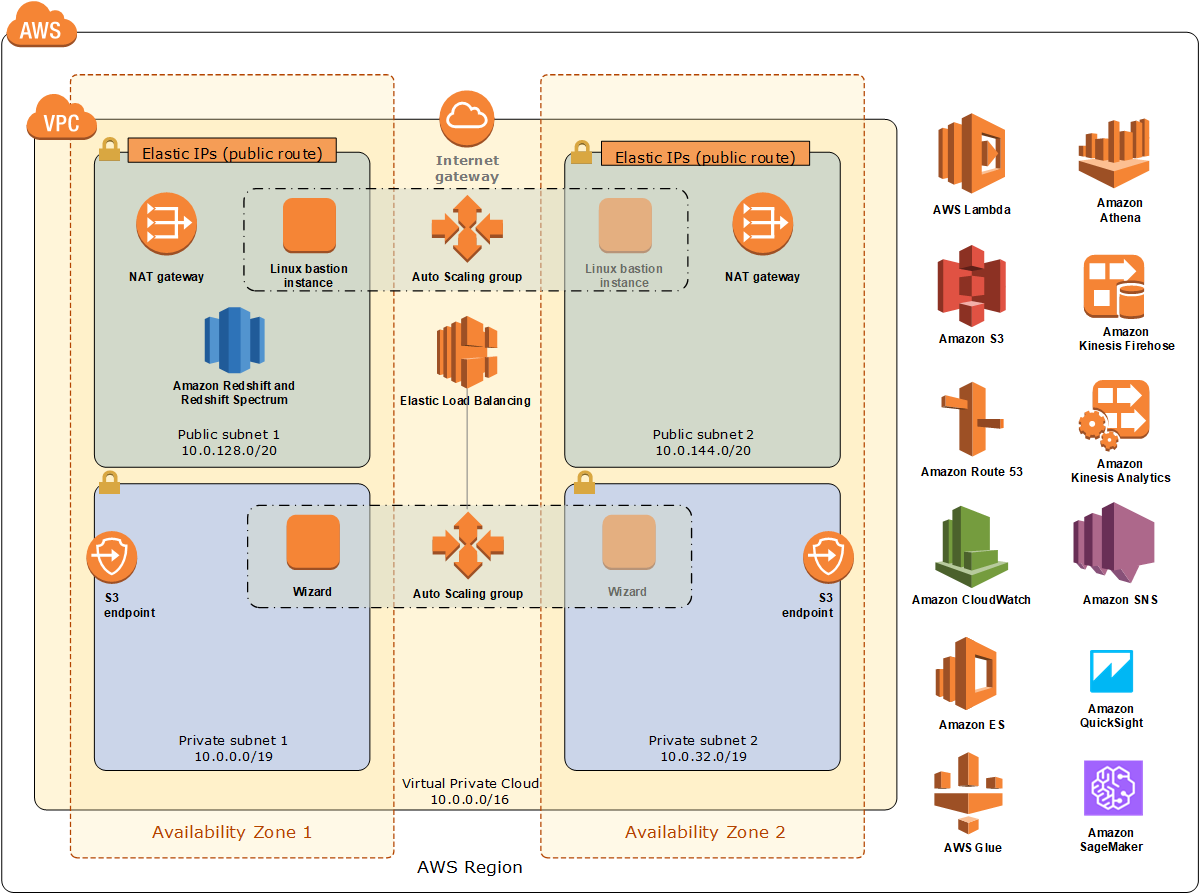
These all bind an organization’s growth to the growth of the appliance provider. However, these systems incur operational overhead, are limited by proprietary formats, have limited elasticity, and tie customers into costly and inhibiting licensing agreements.
AWS DATA LAKEHOUSE UPGRADE
To help with this, customers upgrade their traditional on-premises online analytic processing (OLAP) databases to hyper converged infrastructure (HCI) solutions. Organizational analytics systems have shifted from running in the background of IT systems to being critical to an organization’s health.Īnalytics systems help businesses make better decisions, but they tend to be complex and are often not agile enough to scale quickly.
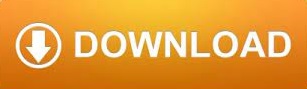